Published on: March 29, 2023 Updated on: February 4, 2024
The Category of AI That Emulates the Way the Brain Works
Author: Lucie Baxter
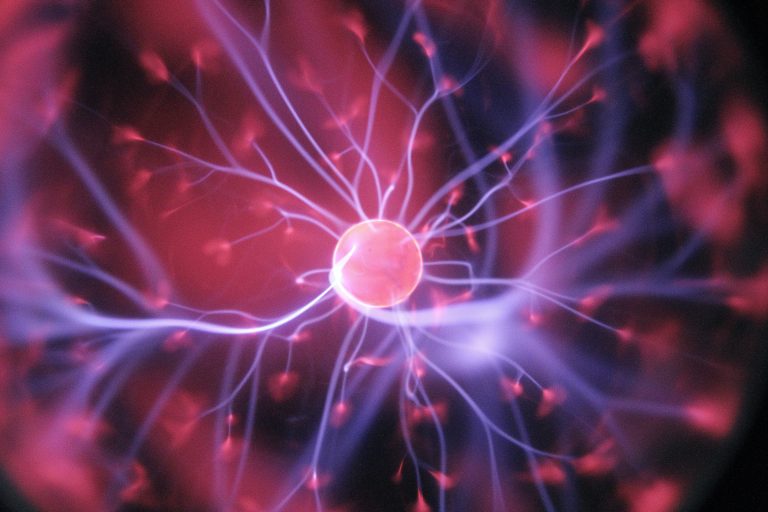
There is nothing more complex and fascinating than the human brain. This hub of intelligence controls more functions than we can even begin to list, and yet its storage capacity is still considered virtually unlimited.
Not too long ago, people would’ve laughed in your face if you told them it was possible to teach a computer the ways of the remarkable organ. However, due to an evolving deep learning (DL) industry and a breakthrough in 2012, neural networks are truly possible.
Naturally, you’re going to be a bit curious. So, in this article, we will help you get to grips with these clever systems and what the future might have in store for us. Let’s get started!
What is a neural network?
A neural network is a method of artificial intelligence (AI) that trains a computer system to process data in a way that imitates a human brain. They are designed to recognize patterns and solve complicated problems through their ability to learn. Cool, right?
But how does it work?
- Data is passed from the input layer to the hidden layer
- Each hidden layer consists of neurons, which is where the data is processed
- The analysis is sent to the third component, the output layer, as an end result
Neurons connect with other neurons to form a network, just as they do inside our heads. The difference is that artificial neural networks are a subset of machine learning (ML) and the backbone of DL algorithms.
Types of neural networks
Separate parts of our brains control each of its unique functions and neurons are responsible for the information systems within them. In the same way, AI researchers are developing different types of neural networks that can solve different problems. Below are the most common variations of these intelligent systems.
- Feedforward neural networks (FNN). Also referred to as an artificial neural network (ANN), these were among the first learning algorithms. Simple but amazing, they are used to perform basic pattern and image recognition.
- Recurrent neural networks (RNN). An extension of the type above, these are much more complicated. Therefore, they are used in natural language processing and speech recognition.
- Convolutional neural networks (CNN). This type is mostly used for computer vision, which allows the machine to find meaningful information in images and videos. So naturally, they are used in object recognition and video analysis.
What is a neural network capable of?
Yes, these artificial intelligence systems do sound like something straight from a science fiction movie. But the concept is certainly not futuristic and unfathomable. Developers continue to push them further, and they are already able to do some remarkable things. In addition to the uses we mentioned above, here are just a few of their valuable skills.
- Intelligent decision-making with limited human assistance
- Goal-seeking analysis for data-driven predictions
- Automation of specific tasks
- Quick processing of datasets
- Can be used in a decision support system (DSS) as data analysis tools
- Problem-solving without needing to be programmed with rules to follow
- Learning trial-and-error through feedback, known as reinforcement learning
Neural networks in the real world
When you combine human intelligence with computer science, the possibilities are endless. Neural networks can be used in the real world, and even in our daily lives.
There are many examples of corporate giants using them in their next big design or project. By integrating AI, they manage to stay ahead of the competition and upkeep their promises of innovation.
Waymo, formally Google’s Self-driving Car Project, is a perfect example of neural networks being implemented for commercial use. This new way of mobility uses AI and machine learning in an attempt to make the roads safer and more accessible.
Not only is it adorable, but it’s clever too. Now a fully realized concept, it is a sight to behold. The intelligent agent uses neural networks to interpret sensor data, allowing it to have a deep understanding of the world around it.
The expert system has even learned to recognize cats, dogs, and when children are about to step off a school bus in real-time.
Neural networks have the capability to reduce the number of car-related crashes and offer greater independence. And that is only the beginning.
AI systems are also being used in industries like finance, security, and medicine due to their capacity for general intelligence.
We know not everyone will have a self-driving car anytime soon, but who knows what will be normal in a few years time? It’s safe to say that neural networks will continue to improve and their potential is immeasurable.
Will they be able to predict the future one day? Since they can’t just yet, only time will tell.
The pros and cons of neural networks
IBM Developer stated that neural networks experienced a “decline, when they failed to meet the unreasonable expectations of their creators”. There are also plenty of debates out there asking if these systems are worth the hype.
Like so many things, they have plenty of pros and cons for you to consider before making a judgment.
Bear in mind that each net is not one-in-the-same. Some are very simple and others are very sophisticated. The advantages and disadvantages won’t always apply, which is something you should be aware of.
However, here is a general list to get you started:
Pros of neural networks | Cons of neural networks |
They can organize data extremely well by categorizing and processing it, which used to require a team of skilled people. | You cannot tell why or how the neural network came up with a particular output. This is difficult to learn from and identify any mistakes. |
If the input changes, they can adapt seamlessly to this and continue functioning under the new instruction. | They can take a lot of time and effort to create and train, especially if your problem or task is complicated. |
Neural networks are used in many industries and contribute to things like chatbots, facial recognition, and clinical diagnosis. | They need a lot of training samples to be able to make generalizations and predictions. |
Neural networks can be effectively used in a wide range of industries, but also aren’t reserved for data scientists. | There are simpler and faster models available to help people, business owners and managers in particular, make decisions (e.g. decision trees) |
They are designed to learn which means they are always improving their results. | Neural networks don’t offer much control to their users, so outcomes can be unpredictable. |
Conclusion
AI’s influence on technology naturally has a huge impact on all of us. It might not be taking over the world in an evil robot sense, and never will for that matter, but it has definitely found its place in the mainstream.
Whether you’re a content creator, student, business owner, or something in between, these scientific discoveries will change everything. That doesn’t have to be a scary thought—ease your anxieties by keeping up-to-date with each impressive development, trend, and breakthrough.
The question remains: how far will neural networks go? No one can say for sure. Google’s AI was the first to pass the Turing test in 2023, so all we can do now is wait and see what’s next.
One thing is guaranteed though. It’s going to be exciting.
There are so many useful AI-powered apps out there that work together to build a mind-blowing technology-driven future. Find the latest and greatest right here at Top Apps.
Lucie Baxter
Lucie is a keen content writer who loves diving into everything tech and AI-related. Since graduating from university, she has been working for a range of diverse companies to continue broadening her writing opportunities.
Recent Articles
Learn how to use advanced search tools, newsletters, and reviews to uncover the perfect AI-focused podcast for you.
Read MoreExplore the top beginner-friendly AI podcasts. Our guide helps non-techies dive into AI with easy-to-understand, engaging content. AI expertise starts here!
Read MoreExplore the features of The AI Podcast and other noteworthy recommendations to kick your AI learning journey up a notch. AI podcasts won’t...
Read More