Published on: June 12, 2023 Updated on: January 24, 2024
AI Solutions for Real World Issues: Saving the World With Tech
Author: Daniel Coombes
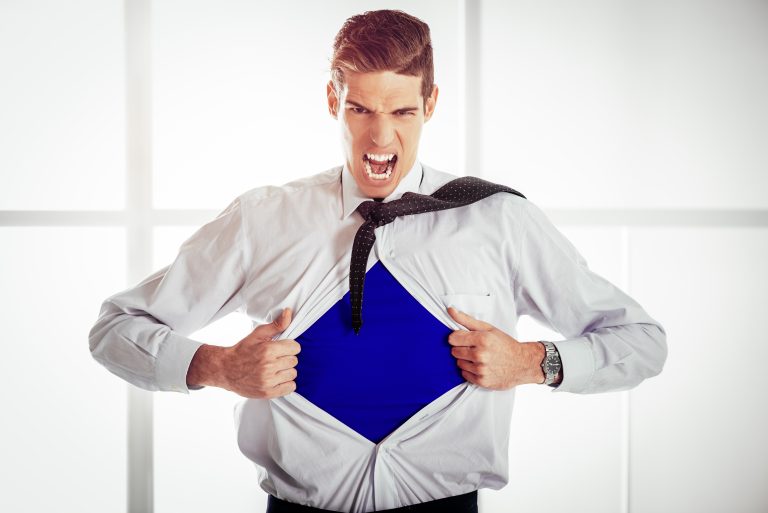
In 2023, nearly every major corporation is using artificial intelligence to some extent to benefit their business model:
- Amazon and other e-commerce brands are using complex algorithms to create tailored-made shopping experiences for their users.
- Social media services are utilizing the power of AI for targeted marketing guaranteed to boost click-through rates.
- Netflix implements a combination of machine learning and data science to recommend your ideal entertainment package.
- Retailers like Walmart and Target use chatbots, similar to ChatGPT, to resolve customer service queries effectively.
- Generative AI uses natural language processing (NLP) to understand user inputs to create high-quality content.
While these use cases are undeniably impressive and beneficial to corporations, it does little to solve real-world problems affecting everyday life.
The world is constantly under threat from extreme poverty, famine, and unrelenting pandemics. In this blog, find out how artificial intelligence is being used to address these complex problems in new and innovative ways.
Three world problems AI can solve
Come with us as we take a look at three of the most pressing issues facing the human race and how AI can resolve them.
1. Poverty
It is no secret that one of the greatest issues facing the world is extreme poverty. Throughout the years, the world has been united to battle this silent killer that has left countless lives in inhumane living conditions.
Charitable events such as Live Aid and Live 8 have undeniably made great strides in providing relief to those in need. Yet there are still 719 million people across the globe battling to survive the harsh reality of poverty.
If you are still struggling to understand the full disastrous extent of world poverty, then take a look at these shocking statistics:
- One of the most devastating consequences of poverty is that 22,000 children die every single day from this preventable cause.
- Since the 2020 pandemic, the number of people living on a daily income of less than $2.15 has reached 700 million.
- Imagine your life without electricity; unfortunately, that is a reality for over 1.6 billion people.
- Poverty may seem like a distant problem in other countries, but it is currently affecting 37.9 million Americans.
One of the biggest challenges facing organizations trying to solve the poverty crisis is identifying the poorest communities.
In developed countries, finding these targets is relatively simple by analyzing census data and household surveys. Unfortunately, in the least developed countries, as seen in the image below, this information is not readily available, meaning that data scientists lack the crucial information needed to identify poor-income areas.
In modern times, scientists have traditionally used night-time satellite imagery to understand the economic layout of the world. This means that the dark areas of the globe are generally underdeveloped countries, based on their energy consumption, while regions that emit light tend to be more developed communities.
However, this basic method is unable to differentiate between near-poverty and absolute poverty. These terms can be defined as:
Near-poverty: This term means a household with an income between 100%-125% of the poverty threshold of $2.15 daily income.
Absolute poverty: This refers to a household that earns below the minimum poverty line.
A sophisticated combination of deep learning and data science is set to revolutionize this inaccurate procedure. Scientists from Stanford University have provided machine learning algorithms with both nighttime and daytime images of underdeveloped countries. The AI system then identified correlating landscape features during the day with areas emitting light at night to locate poverty-stricken areas.
Utilizing this extracted feature data, the algorithm could predict areas of poverty throughout Uganda, Tanzania, Nigeria, Malawi, and Rwanda. Scientists estimated, using available household survey data, that this machine-learning model predicted poverty 81% more accurately than traditional methods.
This type of predictive analytics could have multiple benefits, including:
- Identifying the most poverty-stricken areas and using demand forecasting to identify areas that need more funding.
- Evaluating the effectiveness of antipoverty programs on underdeveloped regions.
- Monitoring the economic well-being of different countries as global dynamics constantly shift.
2. Famine
Chef José Andrés, founder of World Central Kitchen, states:
Food is national security. Food is economy. It is employment, energy, history. Food is everything.
We often neglect to realize how lucky we are to live in a country where food is available in abundance. This basic human right is not met worldwide, with over 828 million people bordering on the verge of famine.
The charity Action Against Hunger states that the following countries are currently the most affected by famine:
- Afghanistan
- Ethiopia
- Nigeria
- Somalia
- South Sudan
- Yemen
Famine is caused by a wide variety of factors, including:
- Climate change: Droughts, floods, wildfires, cyclones, and heat waves will grow in frequency due to climate change. It is estimated that 80 million more people will face starvation by 2050 due to the impact of global warming.
- Conflict: War can drastically reduce the availability of food due to limited production, supply chain shortages, and lack of access to food vendors. It is estimated that 70% of people affected by famine live in war-torn countries.
- Poverty: A lack of sufficient income or living in an underdeveloped region directly coincides with famine. As detailed above, the countries most affected by famine are some of the poorest nations in the world.
- Natural disasters: The impact of natural disasters is truly devastating and affects every aspect of a community, taking many years for an area to fully recover. It is believed that 80% of people affected by famine live in areas prone to natural disasters.
- Displacement: The forced mass migration of people due to violence, human rights violations, climate change, and natural disasters are key causes of malnutrition.
Artificial intelligence has numerous use cases in combating the current food shortage crisis, including:
- Optimization of crop yield: Sophisticated algorithms are analyzing data, including soil composition, weather forecasting, and previous harvests, to improve crop yield. The AI technology can use this data to provide real-time recommendations to farmers about when it is best to water and fertilize their crops.
- New crop varieties: Traditionally, the development of modified crops can take an estimated fifteen years. However, the startup Avalo is able to use machine learning to fully simulate the effects of hundreds of different genomes in two to three years. This means that crop varieties that can withstand harsh environmental conditions can be developed far more efficiently, lowering both cost and time consumption.
- Reducing Food Waste: Shockingly, 2.5 billion tons of food is wasted every single year. Using computer vision and advanced neural networks, TOMRA Sorting Solutions is able to effectively categorize food within the production line into ‘good’ and ‘bad.’ This AI system is also able to offer different uses for food based on its perceived grading. For instance, just because a tomato is unfit to be sold in a supermarket does not mean it cannot be repurposed as an ingredient for ketchup. Data analysis can also help in demand forecasting meaning food is not over or underproduced.
- Transportation: It is believed that about 14% of food waste is created during storage and transportation. AI solutions are able to analyze datasets about traffic, weather forecasts, and road conditions to provide the optimal route for transportation. This enables suppliers to make effective decision-making about route planning, thus ensuring minimal damage to their product.
- Weeding: A major issue within farming is that distinguishing a damaging weed from a young crop is incredibly difficult, resulting in a ‘spray and pray’ approach to herbicide application. Robots, such as LettuceBot, are trained on countless images of healthy sprouts enabling them to effectively identify and eradicate weeds. The automation of this process saves farmers time and money and also minimizes wasted crops. It is estimated that, on average, this technology cut losses by an astounding 90%.
- Early detection: The Nutrition Early Warning System utilizes a combination of both machine learning and data science to locate the areas at risk of famine. Using satellite imagery to identify warning signs of droughts, escalating food prices, and crop failure allows decision-makers to make proactive strategies before a crisis hits.
- Crop disease: Advanced computer vision programs allow farmers to take an image of a potentially diseased crop to identify the infecting ailment. The deep learning algorithm will process data used in training to compare the inputted image to known diseases. This incredible technology will enable farmers to make both effective and informed decisions about treatment, saving countless amounts of crops.
3. Disease
On the 11th March 2020, WHO declared that the Covid-19 outbreak was to be officially classified as a pandemic. This worldwide disaster would sadly go on to claim an estimated 6,883,562 lives and infect over 766,895,075 individuals.
Jacob Lemieux, an assistant professor at Harvard Medical School, states:
‘The interval between pandemics is, cruelly, often just long enough that we forget, and just short enough that they happen repeatedly during our lifetimes.’
Scientists believe that the probability of a new pandemic ravaging the world is at about 2.5% to 3.3% every single year. This may seem like a relatively small figure, but it is important to consider that this frightening statistic would mean there is a 45% to 57% chance of a global catastrophe happening in the next 25 years.
In modern times, pandemics are more likely to be prevalent due to numerous factors, including:
- International travel: An incredible 960 million tourists have traveled internationally in 2022. The booming international tourism industry means that foreign diseases are easily transmitted to new regions.
- Urbanization: It is estimated that over two-thirds of the world’s population will live in urban areas by 2050; this translates to an incredible 7 billion people. The urbanization process will cause overcrowding in unhygienic environments where the disease is able to thrive.
- Climate change: Global warming is causing disease-carrying insects to widen their range. One study predicts that by 2050 two types of dangerous mosquitoes will expand their territory, threatening the well-being of 49% of the world’s population.
- Human-to-animal contact: Zoonotic diseases, animal-borne contagions, are becoming more commonplace as we encroach on many species’ natural environment. This drastically increases the chances of pathogens transferring from animals to humans.
With this information in mind, it becomes evident that the world must be prepared for this disastrous possibility. Bill Gates, the founder of Microsoft, stated:
‘The cost of being ready for the next pandemic is not that large. It’s not like climate change. If we’re rational, yes, the next time, we’ll catch it early.’
Artificial intelligence could be the key to identifying early warning signs, adequately preparing for an outbreak, and minimizing future casualties.
Some examples of how this incredible technology could be used to build a proactive framework to combat future pandemics are:
- Zoonotic: It is now believed that raccoon dogs were the initial cause of the coronavirus pandemic. Scientists estimate that 70% of all emerging diseases come from animal origin. This highlights the importance of understanding and predicting zoonotic pathogens. Using multiple sources of data, analysts are now able to simulate numerous interactions between viruses. This allows scientists to predict which disease and region could be the center of the next outbreak.
- Society: Researchers from the Universitat Oberta de Catalunya and the University of the Balearic Island provided an AI algorithm with mass amounts of historical data in the form of newspapers and gazettes about epidemics. This should enable scientists to predict how pandemics will develop and continually affect society.
- Diagnosis: Teams at MIT and IBM created an image recognition software that was trained on the scans of diseased lungs. The machine could then use multiple sources of patient data to identify cases of coronavirus. It was able to detect 17 out of 25 positive cases that radiologists were unable to determine through traditional means. Another team from MIT trained a machine learning algorithm on countless audio clips of coughs to help detect the differences between healthy and infected patients.
- Drug discovery: The process of discovering new drugs for human consumption is lengthy, taking between 10-15 years. However, artificial intelligence is able to analyze countless compounds in minutes to identify viable options for testing.
- Chatbots: During the pandemic, there were considerable amounts of misinformation about both vaccines and the virus itself. Chatbots have been shown to debunk harmful myths and ease fears about the public health crisis. One study found that 20% of anti-vaxxers changed their mind about vaccines after a conversation with a chatbot.
Problem-solving technology
As the different subsets of artificial intelligence technology continuously evolve, we envision a world where AI can be instrumental in solving real world problems. The meaningful insights that AI technologies can uncover are invaluable to all kinds of issues we face as a society.
This could include:
- Cybersecurity threats
- Terrorism
- Natural disasters
- Criminal activity
- Wildlife conservation
- Pollution
- Climate change
- War and violence
To find out how AI systems are continuously advancing and being implemented to resolve real-world problems keep an eye on our blog.
Daniel Coombes
Daniel is a talented writer from the UK, specializing in the world of technology and mobile applications. With a keen eye for detail and a passion for staying up-to-date with the latest trends in the industry, he is a valuable contributor to TopApps.ai.
Recent Articles
Learn how to use advanced search tools, newsletters, and reviews to uncover the perfect AI-focused podcast for you.
Read MoreExplore the top beginner-friendly AI podcasts. Our guide helps non-techies dive into AI with easy-to-understand, engaging content. AI expertise starts here!
Read MoreExplore the features of The AI Podcast and other noteworthy recommendations to kick your AI learning journey up a notch. AI podcasts won’t...
Read More